COLLATERAL EVENT
A perspective on Artificial Intelligence in Industry and Research
These presentations are not available
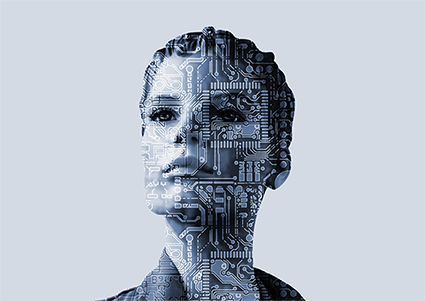
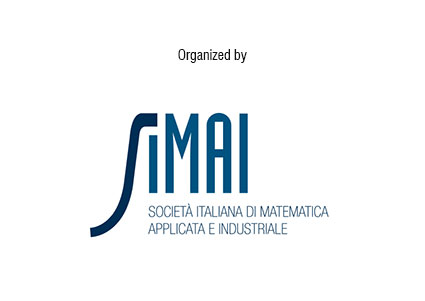
Artificial Intelligence is one of the major drivers of innovation that has emerged in recent years, which has raised many expectations. However, little is known about its applicability and limitations.
Several aspects of the algorithm underlying machine learning and deep neural networks have yet to be fully understood.
This workshop aims to bring together experts from both the academic and the industrial worlds to generate a critical assessment of the prospects for this technology.
The full presentations are not available for this workshop.
Please contact info@caeconference.com if interested in these topics.
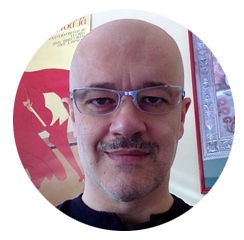
Pierluigi Contucci, Università di Bologna
Artificial Intelligence and machine learning. Challenges and opportunities for mathematicians.
Abstract
Machine learning methods are briefly illustrated and interpreted in mathematical terms together with some of their open problems within the statistical mechanics approach. Some research announcement and their perspectives are formulated together with a broader view of the challenges and opportunities for our community.
Curriculum
Pierluigi Contucci holds a PhD in Mathematics, a MS in Mathematical Physics (ISAS, Trieste) and a Laurea in Physics (University La Sapienza, Rome). His research interests are in Mathematical Physics, Statistical Mechanics and applications (machine learning, data science). He authored more than a hundred papers in international refereed journals, two books and several volumes. Schloessman Award (2000) from the Max Planck Society. Director of the Bologna Unit of the National Institute of High Mathematics, member of Istituto Cattaneo and member of the Science Academy of Bologna. More infos at: http://www.dm.unibo.it/~contucci/cv.html
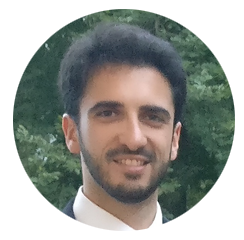
Andrea Bartezzaghi, IBM Research
AI Automation for Scalable AI Adoption.
From automation of NN architecture search, towards the acceleration of the full data science process.
Abstract
AI brings great promise for a new wave of unprecedented productivity across a multitude of industries. Recent AI models have demonstrated extraordinary results in Computer Vision, Natural Language Processing, as well as Business Robotics Automation. However, the complexity of these models has reached enormous levels, with Deep Learning networks often reaching the hundreds of millions of parameters. The traditional approach of human curation and tuning of AI models is clearly not scalable. We will discuss recent solutions proposed by IBM Research in automating neural architecture search, as well as approaches to bring automation towards the acceleration of the full data science process.
Curriculum
Dr. Andrea Bartezzaghi is a Post-Doctoral student in Foundations of Cognitive Solutions. At IBM Research from 2019, he is developing AI-based solutions for the automation of the full data analysis pipeline, from preprocessing of raw data, to network architecture optimization and training. Dr. Bartezzaghi received his B.Sc. and M.Sc. in Mathematical Engineering from Politecnico di Milano (Italy) in 2010 and 2013, respectively. He obtained his Ph.D. in Applied Mathematics from the École Polytechnique Fédérale de Lausanne (EPFL, Switzerland) in 2017. His research interests are in the field of High Performance Computing, Computational Mathematics, Deep Learning, Time-Series Analysis, and Automation of AI.
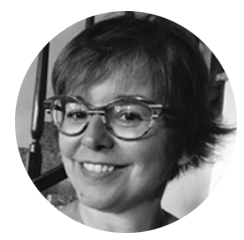
Francesca Perino, MathWorks
Developing Game-Changing Embedded Intelligence
Abstract
The buzz about AI, or artificial intelligence, is deafening. Predictions abound that it will power a massive shift in the roles that computers play in our personal and professional lives:? implementing automated driving functionality, predicting maintenance of industrial equipment, delivering intelligent home health care systems and robots, and more.? But, to get there, teams that combine specialized knowledge, domain expertise, and business objectives must navigate through numerous choices – algorithms, processors, resource location, architectural allocation, communications, and more. While doing so, they must keep their eye on the application that will create the game-changing value for their organization. In this keynote, we look at the promising opportunities and practical challenges of building AI into our systems and services.
Curriculum
Francesca Perino is Senior Application Engineer at MathWorks. She focuses on enabling MathWorks customers to be successful in their adoption and use of MATLAB platform to solve their numerical modelling challenges with cutting-edge technology, tools and methods. She has expertise in data ingestion and processing, software design and application development in MATLAB, distributed computation, big data for enterprise-scale predictive analytics.
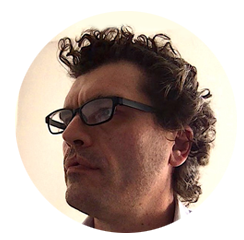
Enrico Busto, CTO @ Addfor
THE RISE OF THE GANs (GENERATIVE ADVERSARIAL NETWORKs).
Approaching the Last Frontier: Machine Dreamse
Abstract
Speaking about Artificial Intelligence, GANs have been defined “the most interesting idea in the last 10 years in Machine Learning”.
GANs give imagination to the machines: they can be used to transform pictures, invent new objects and picture a person that never existed.
Today we'll see how Addfor used GANs to overcome "Algorithmic Discrimination" in biometry by studying how a face recognition camera recognises a person. We will also see an application to Image Restoration for Criminal Investigation.
Curriculum
Enrico Busto has been working since graduation in the field of Artificial Intelligence applied to engineering (actually, in 1995 A.I. was known as "Neural Networks”).
After a 10-years working experience with a leading company, developing mathematical computing software for engineers and scientists, he founded Addfor in 1998.
From the beginning he drove the company focus on selecting, testing and implementing the best available Artificial Intelligence technologies to create innovative solutions, from advanced controls for high performance cars to guidance systems for aerospace applications.
Enrico holds Master Degree of Aeronautical Engineering from Politecnico di Torino and Imperial College London.
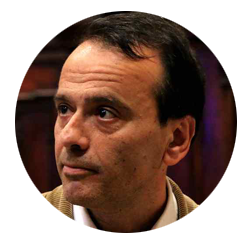
Alessandro Verri, Università di Genova
Artificial Intelligence and Machine Learning: a Heretic View
Abstract
The machine learning of the last few years seems to certify the coming of age of artificial intelligence. At a closer scrutiny, however, the undisputable success of machine learning is confined to a certain class of applications. Walking through research carried out in my group in the last decades, I'll advocate that relevant steps toward the construction of truly intelligent systems can be obtained by developing complex computational models and resorting to the use of sophisticated mathematical tools.
Curriculum
Alessandro Verri born in 1960 received both the Laurea and the PhD in Physics from the University of Genova in 1984 and 1988 respectively. Since 2000 he is Professor of Computer Science with the same University. He has been Visiting Scientist and Professor at the Massachusetts Institute of Technology (Cambridge MA) in 1986, 1988, 1998, 1999, 2001, and 2013, at the International Computer Science Institute (Berkeley CA) in 1992, at the INRIA (Rennes FR) in 1994, and at the University of Heriot-Watt (Edinburgh UK) in 1996.
His scientific interests include learning theory, learning algorithms, biomedical data analysis, and computer vision. He coordinated and coordinates many projects of basic and applied research and technology transfer. He published more than 150 papers on computer vision, modeling of motion perception, learning theory and algorithms, biomedical image analysis, and computational biology. He coauthored a book on computer vision with Emanuele Trucco published by Prentice Hall. According to Google Scholar he received about 12,000 citations and his h-index is 44.
He taught undergraduate and graduate courses on Signal and Control Theory, Information Theory, Introduction to Programming, Computer Architecture, Computer Vision, and Machine Learning. From 1998 to 2001 he taught a graduate course at MIT on Machine Learning. He has been advisor of about a hundred MSc students and 20 PhD students.
With the University of Genova he served as Chairman of the Department of Computer Science from 2006 to 2012, Chairman of the Board of the Department Chairmen from 2008 to 2011, and Director of the Center for Research in Computational Learning from 2006 to 2012. Since 2016 is the Coordinator of the Undergraduate and Master of Science Program in Computer Science.
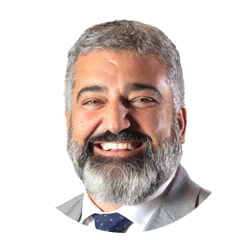
Mattia De Rosa, Microsoft - Cloud and Enterprise Business Group Lead
AI - Applied Intelligence. The use and the promise of AI in the Industry 4.0
Abstract
Artificial Intelligence reached unprecedent level of advancement and applicability in the industry. In this talk we will review the state of the art, some relevant use case and near future scenarios.
Curriculum
Mattia De Rosa is the Director of the Cloud & Enterprise Division looking after the Cloud, Data and AI business on Microsoft Italy. He was previously responsible for Data Platform solutions. Before joining Microsoft, he gained experience in Discovery Reply as Business Unit Manager, in SHS Multimedia Spa as Account Manager with responsibility for managing innovative projects for large customers and, finally, in IBM Italy as Technical Leader in the pervasive computing area.
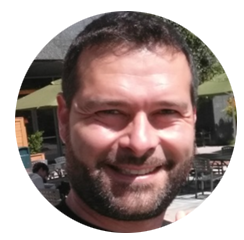
Andrea Gigli, Gamma Lead Data Scientist - BCG
AI adoption in business
Abstract
The talk will give a view of AI adoption across different industries worldwide and summarize the characteristics which differentiate AI Pioneers from AI laggards. Some examples from BCG experience will show how AI can be leveraged to catch new business opportunities.
Curriculum
Andrea Gigli is Lead Data Scientist at Boston Consulting Group with on-the-ground experience in predictive modeling, customer analytics, information retrieval, and optimization. He also leads the development of BCG GAMMA in Italy, Greece, Turkey and Israel.
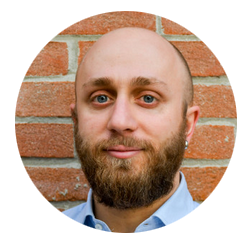
Emanuele Battistello, Cuebiq Data Scientist
Using Machine Learning for multichannel marketing attribution
Abstract
Within Marketing, Attribution modeling is a framework for analyzing which touchpoints, or marketing channels, receive credit for a conversion.
Traditional attribution techniques are mainly rule based, but this approach is beginning to show several limitations.
Rather than using the same set of rules for all marketing campaigns, Cuebiq uses a Data Driven approach, which takes into account the fact that the effectiveness of the same channels can be very different from one marketing campaign to another.
Identifying users exposed to the campaign is a key stepping stone to be able to determine attribution, campaign channels can be online (i.e. web advertising) or "offline" (i.e. out-of-home advertising). Offline channel exposure measurement relies on the availability of location data.
In this speech we will describe how does our Machine Learning based Marketing Attribution Model work and how we leverage our location data, collected from anonymous mobile users, to track customer exposition to offline media channels
Curriculum
Graduated in Mathematical Engineer at Politecnico di Milano with Master Thesis at Chalmers University, Göteborg (Sweden), Data Emanuele Battistello is a data scientist with 5 years of experience. He is focused in applying data mining techniques, doing statistical analysis, and building high quality machine learning systems integrated with our products."